Face Swap Video By Deepfake Old Version
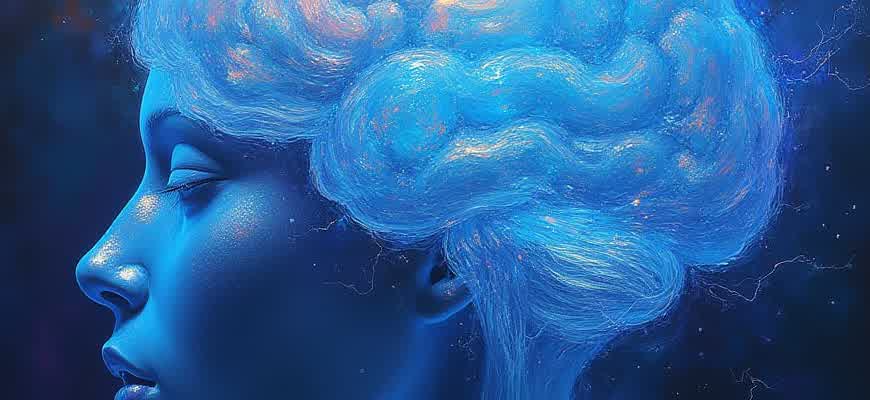
The concept of face swapping through deepfake technology has evolved significantly over the years. In its earlier stages, the tools available for creating these manipulated videos were far more primitive compared to today's advanced models. Below is a comparison between the old and modern versions of deepfake face-swapping technology:
Feature | Old Version | Modern Version |
---|---|---|
Video Quality | Low resolution, visible artifacts | High resolution, almost indistinguishable from reality |
Processing Time | Several hours or days | Minutes to hours with powerful hardware |
Accuracy of Facial Features | Distorted or unnatural facial expressions | Perfect facial integration, real-time motion tracking |
"In the early days of deepfake technology, face swapping was a time-consuming and error-prone process, often leaving noticeable signs of manipulation."
One major limitation of the earlier iterations was the inability to seamlessly blend the facial movements and expressions with the original video. Often, the face would appear stiff or misaligned with the person's movements. These imperfections were particularly noticeable in videos with fast motion or complex lighting conditions.
Despite these drawbacks, the old deepfake models paved the way for the advancements we see today. They were the first steps toward the highly sophisticated systems capable of generating hyper-realistic face swaps in real-time. The progress in deepfake technology continues to raise both technical and ethical questions, as the potential for misuse increases with each improvement.
Creating a Face Swap Video Using the Deepfake Old Version Tool
The Deepfake Old Version tool offers a simple yet powerful way to create face swap videos by using machine learning algorithms. This tool allows you to swap the faces of people in a video while maintaining a high level of realism. However, it’s important to understand the process and requirements before starting. Below is a step-by-step guide to help you get started with face swapping using this tool.
First, you will need to gather the right resources. This includes a high-quality video and clear images of the faces you wish to swap. The more data you provide to the tool, the better the final result. Now, let’s look at the procedure involved in creating a face swap video.
Step-by-Step Guide
- Download and Install Deepfake Old Version Tool: Begin by finding and downloading the specific version of the tool. Make sure to use the version that matches your system requirements for optimal performance.
- Prepare Video and Images: Ensure you have a video file and clear images of the faces you want to swap. High resolution and good lighting in the images will improve the quality of the face swap.
- Upload Video to the Tool: Open the Deepfake tool and upload the video that you want to modify.
- Input Face Data: Upload the images of the faces you wish to swap into the software. The tool will need to process these faces in order to map them onto the video.
- Adjust Settings: Make any necessary adjustments to the settings, such as alignment or face features, to ensure the faces match well with the video.
- Run the Algorithm: Once all the data is in place, run the tool's algorithm. This process may take some time depending on the video length and the complexity of the faces being swapped.
- Review and Edit: After the tool completes its work, review the video. Make any final edits, such as smoothing out transitions or correcting any mismatched frames.
- Export Video: Finally, export the face-swapped video to your desired file format.
Important Notes
Remember that creating face-swapped videos using deepfake technology may have ethical implications. Always seek permission from the individuals whose faces you are swapping and ensure the content complies with relevant laws and guidelines.
Requirements for Optimal Results
Requirement | Details |
---|---|
Video Quality | High resolution with good lighting for better face mapping. |
Face Image Quality | Clear and well-lit facial images ensure accuracy in the swap. |
System Requirements | Ensure your computer meets the minimum requirements for the tool. |
Understanding the Limitations of Deepfake Technology in Face Swap Videos
Deepfake technology has made significant progress in the creation of face swap videos, allowing for seemingly realistic alterations to video content. However, while these tools have gained popularity, they are not without their limitations. The older versions of face swap technology, despite impressive results, struggled with several key issues that hindered their effectiveness in creating seamless and convincing videos.
One of the most notable challenges with early deepfake systems was their inability to perfectly replicate facial expressions and nuances. As a result, these videos often exhibited unnatural movements or inconsistencies when the swapped faces were involved in dynamic actions, leading to a noticeable disconnect between the individual and their new face. Additionally, the technology relied heavily on large datasets and powerful processing, which was not always accessible for everyday users.
Key Limitations of Early Face Swap Technology
- Inconsistent facial movements: Early deepfake models often struggled with reproducing subtle expressions like blinking, eyebrow movements, or mouth shifts.
- Poor resolution and artifacts: Low-quality inputs or insufficient data would result in blurry, pixelated images and visible artifacts around the face, making the swap easily noticeable.
- Facial alignment issues: A mismatched alignment between the face and the body could result in awkward angles or unrealistic postures that disrupted the video’s flow.
- Limited facial data: Older systems lacked the depth of facial features needed for more complex swaps, especially in scenarios with multiple people or crowded settings.
Key Factors Impacting Early Deepfake Performance
- Data Availability: Deepfake tools require vast amounts of data to accurately recreate faces. Older models lacked access to such large datasets, limiting their accuracy.
- Processing Power: Generating high-quality deepfake videos demanded significant computational resources, which were not accessible to all users, leading to subpar results for many.
- Model Complexity: The early algorithms were not sophisticated enough to handle nuanced aspects of facial recognition, such as lighting variations, shadows, and background changes.
"While deepfake technology has evolved rapidly, early versions were far from perfect and still carried noticeable flaws in their output, particularly in face swap scenarios."
Comparison of Early vs. Modern Face Swap Technology
Aspect | Early Deepfake Technology | Modern Deepfake Technology |
---|---|---|
Facial Expression Accuracy | Limited; often unnatural movements | Highly realistic; smooth transitions |
Resolution and Artifacts | Visible pixelation and artifacts | High resolution with minimal artifacts |
Facial Alignment | Frequent misalignment and unnatural posture | Precise alignment, even with movement |
Processing Power | High computational demand, limited access | More accessible tools with efficient processing |
Optimizing Video Quality for Realistic Face Swaps with Deepfake Old Version
Achieving high-quality face swaps with older deepfake versions requires a keen understanding of the technical aspects that affect the final video output. While newer algorithms have made significant strides in facial recognition and synthesis, older versions still provide substantial tools for realistic transformations if used correctly. Fine-tuning key settings and utilizing specific techniques can significantly improve the quality of face-swapped videos, reducing artifacts and enhancing the realism of the facial features.
The core elements of optimizing video quality when working with older deepfake models involve a combination of data input quality, model training, and post-processing adjustments. The process may take longer compared to modern systems, but with the right methods, users can still achieve convincing results. Here, we will explore the main factors that contribute to improving face swap realism in deepfake videos created with older tools.
Key Factors to Enhance Deepfake Video Realism
- High-Quality Source Footage: Starting with clean, high-resolution source images and videos is critical. The better the input material, the more detailed and realistic the output will be.
- Model Tuning: Properly training the model with diverse datasets and fine-tuning its parameters can reduce distortion and improve facial feature alignment.
- Frame Consistency: Maintaining smooth transitions between frames ensures that facial movements and expressions are consistent throughout the video.
Optimization Techniques for Deepfake Face Swaps
- Preprocessing: Normalize and align facial images to create consistent input data, reducing mismatch in facial features during the swap.
- Model Adjustment: Use older deepfake versions' settings to enhance the blending of facial textures with the target video, ensuring that skin tones and lighting match.
- Post-Processing: Apply software tools for color correction, motion smoothing, and artifact removal after the face swap to further refine the output.
Challenges and Solutions
Even with optimal settings, older deepfake models may still produce imperfect results, such as visible seams, unnatural eye movements, or incorrect lighting. However, these issues can be minimized by experimenting with different datasets, adjusting learning rates, and using more advanced post-processing techniques to blend the face seamlessly with the original scene.
Table of Deepfake Model Settings
Setting | Description | Optimization Tips |
---|---|---|
Resolution | Higher resolution leads to better detail in the face swap. | Use the highest available resolution for source images and videos. |
Learning Rate | Controls the speed at which the model learns to make adjustments. | Use a slower learning rate for more detailed face feature alignment. |
Iterations | Number of times the model adjusts the face swap. | Increase iterations to improve facial accuracy, but avoid overfitting. |
Common Mistakes When Using Deepfake for Face Swap Videos and How to Avoid Them
Creating realistic face swap videos using deepfake technology can be a highly rewarding experience, but there are common pitfalls that can drastically reduce the quality of the output. Understanding these issues and taking steps to address them can make the difference between an impressive and a flawed video. In this guide, we will discuss key mistakes that are often made when applying deepfake technology for face swapping, and how to avoid them.
One of the most frequent errors involves poor alignment of facial features. When the original face and the target face are not properly aligned, the resulting video can appear unnatural or distorted. In addition, issues like improper lighting, low-quality source material, and insufficient training data can all contribute to a less-than-optimal outcome. Below are some of the common problems and practical tips on how to avoid them.
1. Inaccurate Facial Alignment
- Ensure the faces are positioned in similar orientations to reduce distortion.
- Use advanced facial landmark detection tools to accurately track facial features.
- Always verify the alignment during each frame for consistency across the video.
2. Low-Quality Input Materials
- Use high-resolution images and videos for both the original and target faces.
- Ensure consistent lighting and background conditions in the source material.
- When working with low-quality sources, consider enhancing them using image-editing tools before running them through the deepfake model.
3. Insufficient or Unbalanced Training Data
- Gather a variety of images from multiple angles to provide the model with diverse data for better face replication.
- Ensure that both faces (the original and the target) have enough data for the deepfake algorithm to learn from.
- Double-check the training set for any biases or inaccuracies that may affect the final video quality.
Important Tip: Consistently monitoring the deepfake model’s output and making adjustments as necessary will ensure a more realistic final product.
4. Overuse of Post-Processing Filters
- Avoid excessive reliance on post-processing effects that can alter the face too much.
- Instead, focus on improving the quality of the input video before resorting to filters.
- Test different post-processing techniques on a small portion of the video first, to avoid ruining the overall effect.
5. Ignoring Frame Consistency
Issue | Solution |
---|---|
Frame-to-frame inconsistencies in facial motion | Ensure smooth transitions between frames by adjusting the deepfake model’s parameters to maintain continuity. |
Unnatural facial expressions or unnatural syncing | Review the model’s output and apply corrective tweaks to enhance facial synchronization. |
How to Manage Facial Movements and Expressions in Deepfake Videos
When creating deepfake videos, one of the most challenging aspects is replicating accurate facial expressions and movements. These features are essential for ensuring the realism of the altered content. Manipulating facial muscles, especially in response to various emotional states or speech patterns, can make or break the believability of the result. An accurate recreation of movements requires advanced algorithms and a deep understanding of human facial anatomy.
The technology behind deepfakes works by analyzing the underlying features of the original video and transferring them to a target face. However, it’s not just about simply swapping faces. The new face must match the subtle, dynamic movements of the original, such as blinking, lip movements, and muscle tension around the eyes and mouth. If these movements are not carefully handled, the deepfake video can appear unnatural or “off,” breaking the illusion.
Key Considerations for Facial Movement Replication
- Emotion Expression: The facial movements must reflect the underlying emotions, such as surprise, happiness, or sadness. Subtle changes in eyebrow position, mouth corners, and eye openness play a significant role.
- Speech Synchronization: Lip movements must be synced with the audio to ensure coherence between what is being said and how the face moves.
- Eye Movement: Eyes should maintain realistic motion patterns, such as following the speaker or reacting to the environment in the scene.
- Subtle Micro-Movements: Small muscle adjustments, such as the twitch of an eyebrow or a slight tilt of the head, are crucial for achieving natural realism.
Techniques for Handling Facial Movements
- Data Augmentation: Enhance the dataset by adding variations of facial expressions to train the model more effectively.
- Real-Time Tracking: Implement real-time facial tracking to ensure that the transferred expressions follow the subject's movements accurately during video playback.
- Feature Layering: Combine different layers of facial expressions to match dynamic scenes more seamlessly, ensuring the transitions are not jarring.
- Deep Learning Models: Use neural networks trained on large datasets of facial expressions and movements to ensure more natural and contextually appropriate responses.
Common Pitfalls to Avoid
Issue | Impact | Solution |
---|---|---|
Unnatural Eye Movement | Creates an eerie or robotic feel. | Ensure proper eye tracking and organic movement with external model corrections. |
Poor Lip Sync | Disrupts the illusion of speaking naturally. | Implement automatic lip sync algorithms to adjust for timing mismatches. |
Over-Exaggerated Facial Expressions | May make the video appear comical or less convincing. | Limit the expression intensity to match the original video context. |
Accurately replicating facial expressions in deepfake videos requires a balance of data processing, emotional intelligence, and real-time movement tracking. Without these, the final product risks appearing too artificial or inconsistent.
Improving the Sync Between Voice and Face in Deepfake Videos
Deepfake technology has made remarkable strides in recent years, allowing for more seamless and convincing face-swapping in videos. However, one of the ongoing challenges is achieving a high level of synchronization between the voice and the face. This is crucial for creating a believable and immersive experience, as discrepancies between the two can quickly break the illusion. The need to align the movements of facial muscles with the audio is an essential component in producing more realistic deepfake videos.
To address this issue, several methods have been explored, including advances in machine learning algorithms, improved motion tracking, and enhanced audio-visual integration. A significant part of this effort focuses on making sure that the lip movements and expressions match the rhythm and tone of the speech, providing a more natural and convincing outcome.
Techniques for Enhancing Sync
- Audio-Driven Lip Syncing: Using audio features to guide the facial movements ensures the lips match the phonemes and rhythm of the speech.
- Facial Motion Capture: Tracking the user’s original facial expressions more accurately helps replicate those movements in the deepfake model.
- AI-Based Speech Synthesis: This technology generates lip movements that are more naturally synced with the voice, improving realism.
- Optimized Neural Networks: Deep learning models are trained with vast datasets to better predict and replicate nuanced facial movements in real-time.
Challenges and Solutions
"The key to improving the sync between voice and face in deepfake videos lies in a better understanding of how speech affects facial expressions."
- Challenge: Inconsistent lip sync during rapid speech transitions.
- Solution: Using high frame-rate models that process detailed facial movements at a granular level.
- Challenge: Inaccurate eye movements and other facial expressions not matching the voice tone.
- Solution: Integrating additional data inputs, such as emotional context or environmental factors, into the deepfake model.
Key Considerations
Factor | Importance | Improvement Method |
---|---|---|
Audio Quality | High-quality audio is crucial for accurate syncing. | Use of noise reduction algorithms and clear sound recordings. |
Facial Expression Mapping | Ensures realism in the deepfake output. | Enhanced motion capture and improved deep learning techniques. |
Real-Time Processing | Allows for seamless interaction between voice and facial movements. | Optimization of neural networks for faster processing speeds. |
How to Choose the Right Source Material for Face Swap Videos
When creating face swap videos using deepfake technology, the quality of your source material plays a crucial role in the final result. The wrong choice of images or video footage can lead to a less convincing and distorted output. To ensure the best results, it is essential to focus on specific characteristics when selecting your source material. This includes considering factors such as lighting, angles, and facial expressions of the subjects involved.
Additionally, understanding the resolution and quality of the source media is vital. Higher resolution images or videos allow for better details and more seamless integration of the face swap. This section will discuss the key factors to consider in choosing the ideal source content for a successful face swap experience.
Key Considerations for Selecting Source Material
- Resolution: High-resolution images or videos provide more detail, which is essential for achieving a natural and realistic face swap. Low-resolution media often results in visible artifacts and blurring.
- Lighting Conditions: Consistent and natural lighting is vital for the face swap to blend well. Strong shadows or artificial lighting can create unrealistic contrasts, making the swap more noticeable.
- Facial Expressions: Try to choose footage where the subject's face is neutral or in a relaxed state. Extreme expressions can distort the alignment of facial features in the swap.
- Angle and Orientation: Opt for videos or images where the face is clearly visible and captured from a frontal or slightly angled position. Side views or tilted faces complicate the swap process.
Tips for Finding Ideal Source Content
- Look for videos or images with clear, unobstructed views of the subject’s face.
- Ensure that the camera is steady, as movement can cause misalignment in the swap.
- Choose footage with balanced lighting, avoiding backlighting or extreme contrasts.
Important Technical Details
Factor | Ideal Conditions | Possible Issues |
---|---|---|
Resolution | High (1080p or higher) | Low resolution leads to pixelation |
Lighting | Soft, even lighting | Harsh lighting creates unnatural shadows |
Facial Expressions | Neutral, relaxed | Exaggerated expressions distort the face swap |
Always prioritize high-quality source material to maximize the realism of your face swap video. Proper lighting, resolution, and neutral facial expressions are the key components for achieving a convincing result.