Deepfake Generator Bot
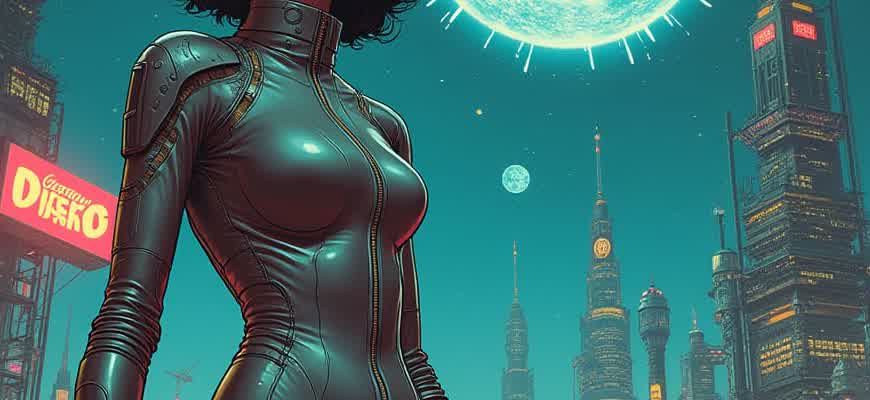
Deepfake creation bots have emerged as powerful tools in the world of artificial intelligence, enabling users to generate highly convincing altered media. These bots leverage deep learning algorithms to create realistic video, audio, and image manipulations, making it increasingly difficult to distinguish between authentic and fabricated content. Below are some of the key aspects of this technology:
- AI-Powered Technology: The foundation of deepfake bots is neural networks that are trained on large datasets of images, audio, or video clips. This allows the bot to replicate facial expressions, voice tones, and movement with remarkable precision.
- Applications: From entertainment and advertising to malicious activities like misinformation campaigns, deepfake bots have found a wide range of uses.
- Legal and Ethical Concerns: The rise of these technologies has sparked debates over privacy, security, and accountability in digital media.
"The technology behind deepfake bots is advancing rapidly, raising serious questions about its implications for society, from media integrity to personal security."
Despite their impressive capabilities, the use of deepfake bots is not without risks. The following points highlight the concerns surrounding their use:
- Privacy Violations: Deepfake bots can manipulate videos and images of individuals without their consent, leading to potential violations of privacy rights.
- Disinformation: These bots can easily be used to create fake news, mislead the public, or defame individuals or organizations.
- Countermeasures: Various technologies, including AI detection tools, are being developed to identify deepfakes and reduce their impact on society.
As the technology continues to evolve, so too does the need for comprehensive strategies to address its potential misuse.
Customizing Output: How to Fine-Tune the Deepfake Quality
Creating high-quality deepfakes requires not only a robust generation model but also the ability to fine-tune various parameters to achieve desired results. Fine-tuning refers to adjusting the model settings and input data to improve output realism, fluidity, and visual coherence. It is a critical step for developers and users aiming to optimize the quality of their deepfake videos or images. The process involves tweaking several elements like resolution, facial landmarks, and frame synchronization to ensure that the deepfake looks as lifelike as possible.
To effectively enhance the deepfake output, there are a few key parameters that need careful attention. Below are several methods and factors to consider when adjusting the quality of generated deepfakes. Fine-tuning is both an art and a science, requiring both technical skills and creative insight to reach the best results.
Key Parameters to Adjust for Optimal Deepfake Output
- Resolution: Increasing resolution helps generate sharper images with more details, resulting in more realistic output. However, this may also require more computational resources.
- Frame Rate: Consistent frame rates across all video segments are crucial for a smooth and fluid deepfake. Varying frame rates can lead to visual inconsistencies.
- Lighting and Shadow Effects: Ensuring that lighting in the source and target videos matches closely can significantly enhance realism. Any discrepancies in shadows or light angles can make deepfakes look artificial.
Adjusting Model Parameters: A Step-by-Step Guide
- Step 1: Start by collecting high-quality, well-lit data for both the source and target subjects. This ensures the model has a solid base to work from.
- Step 2: Choose an appropriate face swap method (e.g., facial landmarks or generative adversarial networks) depending on the complexity of the video.
- Step 3: Fine-tune the model by adjusting hyperparameters such as learning rate, training steps, and batch sizes to control the quality and speed of generation.
- Step 4: Perform post-processing like color grading, noise reduction, or artifact removal to further improve visual quality.
“The quality of the output highly depends on the balance between resolution, frame rates, and model adjustments. A deepfake is only as good as the dataset and fine-tuning methods applied during creation.”
Factors to Monitor During Fine-Tuning
Factor | Impact on Quality | Suggested Settings |
---|---|---|
Face Alignment | Ensures proper placement of facial features | 90-100% accuracy |
Resolution | Sharpness and detail of the deepfake | 1080p or higher |
Training Time | Influences smoothness and realism | Several days depending on model complexity |
Optimizing Speed and Performance for Large-Scale Deepfake Creation
Creating deepfake content at a large scale demands highly efficient systems capable of handling massive datasets and complex algorithms. The performance of deepfake generators largely depends on how well the software is optimized for both processing speed and computational power. Key factors influencing this optimization include the choice of hardware, software architecture, and parallelization techniques that can significantly improve the overall efficiency of the system.
When working with large-scale deepfake production, several strategies can be implemented to minimize processing time and maximize the quality of generated content. By understanding the core components that affect performance, developers can ensure that the system runs smoothly, even under high demand.
Key Techniques for Optimizing Performance
- Hardware Utilization: Leveraging GPUs and specialized hardware accelerators (e.g., TPUs) can drastically improve performance compared to CPU-based processing.
- Data Preprocessing: Efficient data handling, such as image resizing and format conversion, can reduce computational overhead during training and generation.
- Batch Processing: Implementing batch processing techniques allows the system to process multiple deepfake instances in parallel, reducing overall time per operation.
- Distributed Computing: Using cloud-based infrastructure or multiple servers to divide workloads can significantly enhance processing speed and scale.
Performance Metrics and Analysis
To evaluate the success of optimization efforts, it is essential to track specific performance metrics. This allows for fine-tuning of the system and ensures it meets both speed and quality requirements.
Metric | Significance | Optimization Techniques |
---|---|---|
Processing Time | Time taken to generate a single deepfake | GPU acceleration, batch processing |
Image Quality | Clarity and realism of the generated output | Advanced neural networks, hyperparameter tuning |
Throughput | Number of deepfakes generated per unit of time | Parallel processing, distributed computing |
Note: While optimizing for speed, it's crucial to ensure that quality does not suffer significantly, as the balance between these two factors defines the overall success of the deepfake generation process.
Addressing Ethical Concerns: Safe Usage of Deepfake Technology
Deepfake technology has become increasingly accessible, offering users the ability to create highly realistic digital manipulations of video and audio. While this innovation brings potential for creative applications, it also raises significant ethical concerns. The risk of misuse has sparked debates about the balance between freedom of expression and the protection of individuals' privacy, reputation, and security. It is essential to understand how deepfake technology can be used safely and responsibly, ensuring that it does not harm individuals or society at large.
To mitigate the dangers associated with deepfakes, developers, regulators, and users must collaborate to create guidelines and safeguards that promote ethical use. The following strategies can help address these concerns:
Key Principles for Safe Usage
- Transparency and Disclosure: It is crucial to disclose when content is manipulated to avoid misleading audiences.
- Consent: Ensure that individuals whose likenesses are used have given explicit permission for the creation of deepfakes.
- Accountability: Establish legal frameworks to hold creators accountable for harmful or malicious deepfake content.
Practical Guidelines
- Use for Creative Purposes: Focus on using deepfakes for artistic, educational, or entertainment applications, where it does not exploit or harm real people.
- Develop Detection Tools: Invest in technology that can identify deepfakes, which will help protect individuals and businesses from fraudulent content.
- Respect Legal Boundaries: Follow existing laws related to defamation, harassment, and intellectual property to avoid creating content that violates others’ rights.
"Deepfake technology should be used responsibly, with an emphasis on transparency, respect for privacy, and adherence to ethical guidelines."
Possible Consequences of Misuse
Type of Misuse | Potential Impact |
---|---|
Political Manipulation | Undermines trust in media, spreads misinformation, and manipulates public opinion. |
Identity Theft | Invades privacy, damages personal reputations, and facilitates fraudulent activities. |
Harassment and Defamation | Causes emotional distress, damages reputations, and leads to legal consequences. |