Deep Fake Voice Creator
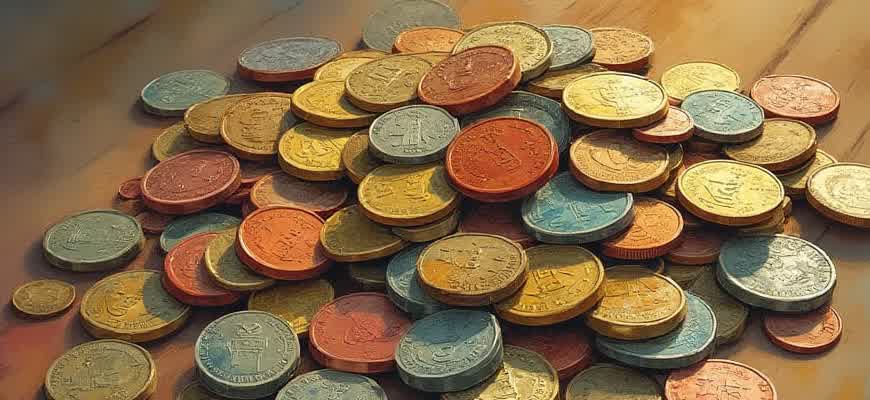
Deep fake voice technology refers to the use of artificial intelligence to synthesize or manipulate speech, making it sound as if a person is speaking when they are not. These systems rely on advanced machine learning techniques to analyze and replicate the nuances of human speech, including tone, pitch, and cadence. This technology has found applications in various fields, from entertainment to cybersecurity, with both positive and negative implications.
Key Features of Deep Fake Voice Technology:
- Ability to mimic voices of specific individuals with high accuracy.
- Generates speech in various languages and accents.
- Can alter tone, emotion, and speed of speech to suit different contexts.
Applications:
- Entertainment industry for voiceovers or recreating voices of deceased actors.
- Security concerns, such as impersonating individuals for fraudulent activities.
- Assistive technologies, like generating personalized voices for those with speech impairments.
"The ethical challenges surrounding deep fake voice technology are significant, as it can be used to deceive or manipulate people, leading to serious consequences in areas like politics, law enforcement, and personal security."
Table 1: Comparison of Deep Fake Voice Tools
Tool Name | Features | Target Audience |
---|---|---|
Descript | Text-to-speech, voice cloning, high-quality audio output | Content creators, podcasters |
Lyrebird AI | Real-time voice cloning, emotion-based modulation | Advertisers, film studios |
Respeecher | Voice replication for media, film, and video production | Media production teams, game developers |
How to Generate Realistic Voices Using Deep Fake Technology
Deep fake technology has become an advanced tool for creating synthetic voices that can closely mimic human speech. By leveraging machine learning algorithms, this technology allows users to generate audio that sounds remarkably natural and indistinguishable from a real human voice. The process relies on training neural networks with vast amounts of voice data to learn speech patterns, accents, intonations, and more. These networks then synthesize new audio based on the learned models, making it ideal for applications ranging from entertainment to security.
To generate high-quality deep fake voices, several steps must be followed, including data collection, model training, and fine-tuning. In this article, we will discuss the key components involved in producing authentic-sounding synthetic voices using deep fake techniques.
Steps to Create a Realistic Voice with Deep Fake Technology
- Data Collection: The first step is gathering a large dataset of voice recordings. This data should contain various emotions, speech patterns, and accents to give the model a comprehensive understanding of different speaking styles. The more diverse the dataset, the more accurate and versatile the generated voice will be.
- Preprocessing and Annotation: Clean the audio data by removing noise and irrelevant sounds. Annotating the dataset with appropriate labels, such as pitch, speed, and tone, helps the model understand the nuances of speech.
- Training the Model: Use machine learning frameworks to train neural networks with the preprocessed data. This involves feeding the data into the model, which adjusts its parameters to generate voice samples that resemble the target voice.
- Fine-Tuning: Fine-tuning is crucial to refine the generated voice. After initial training, adjustments are made to improve the naturalness and accuracy of the voice. This step may require additional datasets or manual corrections to eliminate inconsistencies.
Key Technologies Behind Voice Generation
- Text-to-Speech (TTS) Systems: These systems convert written text into speech. When combined with deep learning, TTS can create voices that sound highly realistic by simulating human-like intonation and rhythm.
- Voice Cloning: Voice cloning technology creates a synthetic voice that mimics a specific individual’s speech. This is done by analyzing a person's voice and using their unique vocal features to replicate their speech patterns.
- Generative Adversarial Networks (GANs): GANs are used to create more sophisticated and lifelike synthetic voices. By employing two neural networks that compete against each other, GANs can generate audio that is more convincing and difficult to distinguish from real speech.
Important Considerations
Ethical Concerns: As with any technology, the use of deep fake voices raises ethical questions, particularly around privacy, consent, and misuse. It is essential to use this technology responsibly to prevent harmful outcomes, such as fraud or identity theft.
Stage | Description |
---|---|
Data Collection | Gathering diverse voice samples from various speakers. |
Preprocessing | Cleaning and labeling the voice data for training. |
Model Training | Training the neural network with the prepared data. |
Fine-Tuning | Improving the model’s accuracy and naturalness. |
Step-by-Step Process for Setting Up Your Deep Fake Voice Generator
Creating synthetic voices using deep fake technology requires a series of steps to ensure accuracy and quality. This process typically involves choosing the right tools, setting up your environment, and training the model to replicate a specific voice. Below is a detailed guide to help you get started with generating artificial voices that sound indistinguishable from real ones.
Before diving into the technical details, ensure that you have all the necessary software, hardware, and voice data. Setting up a deep fake voice generator often requires advanced knowledge of machine learning and audio processing. Below, we outline a simplified guide to walk you through the essential steps of configuration and use.
Step 1: Choose a Deep Fake Voice Tool
Select a reliable deep fake voice generation tool. Popular platforms offer both cloud-based and local options for voice cloning. Make sure to choose a tool that supports high-quality audio generation and has strong community support.
- Check if the tool offers pre-trained models or requires you to train from scratch.
- Verify compatibility with your operating system and hardware.
- Review the pricing plans and available features.
Step 2: Prepare the Audio Dataset
To achieve the best results, you need a dataset of clean audio samples from the target voice. This dataset should cover a variety of tones, pitches, and emotions.
- Record high-quality voice samples in a quiet environment.
- Ensure the audio files are in a supported format, such as WAV or MP3.
- Organize the samples based on the desired phrases or speech patterns.
Step 3: Configure the Environment
Next, configure your local environment for model training. This involves setting up the necessary dependencies, such as Python libraries, machine learning frameworks, and audio processing tools.
- Install required software like TensorFlow, PyTorch, or other deep learning frameworks.
- Set up a GPU environment to speed up model training.
- Ensure the system has adequate memory and processing power.
Important: Make sure to check for any system requirements or prerequisites before starting the setup.
Step 4: Train the Model
Once everything is set up, you can start the training process. The model will learn to generate the target voice by analyzing the dataset and extracting patterns in tone and speech dynamics.
Task | Details |
---|---|
Model Type | Choose between voice synthesis or transfer learning models. |
Training Duration | Training time varies depending on the size of the dataset and the power of your machine. |
Step 5: Generate the Synthetic Voice
After training, you can now use the deep fake voice generator to produce speech. Provide text inputs, and the system will generate audio with the target voice.
- Adjust settings for pitch, speed, and emotion if necessary.
- Test and refine the voice generation to match your expectations.
Tip: Always evaluate the generated voice on different types of text to ensure versatility and quality.
Customizing Voice Tone and Accent for Different Use Cases
When developing synthetic voices for various applications, adjusting the tone and accent is crucial for achieving a natural and engaging interaction. Different use cases require distinct characteristics from a voice, such as professional tone for customer service, friendly tone for entertainment, or authoritative tone for instructional content. Tailoring these elements ensures that the voice aligns with the intended purpose and resonates with the target audience.
Accent customization is equally important, particularly in multilingual applications or content aimed at diverse geographical regions. Whether you’re designing a voice for a global product or catering to a specific locality, accent plays a significant role in making the synthetic voice more relatable and effective in communication.
Adjusting Voice Tone
Voice tone refers to the emotional quality of the speech, which can drastically impact the listener’s perception and engagement. Some of the key tones include:
- Professional: Suitable for corporate environments or technical explanations.
- Casual: Ideal for conversational apps, personal assistants, and social media content.
- Empathetic: Used in customer service or healthcare applications where emotional support is crucial.
- Excited: Best for advertisements, promotional content, or interactive experiences.
Each of these tones can be achieved by adjusting the pitch, speed, and modulation of the voice model. For example, a professional tone may have a slower pace and a controlled pitch, while an excited tone may be faster and more dynamic.
Choosing the Right Accent
Accents enhance the authenticity and local relevance of a voice. Depending on the region or target audience, the following accents may be required:
- Standard American English: Commonly used in global products, as it's widely understood.
- British English: Popular for formal and educational content, often used in high-end branding.
- Australian English: Distinct and friendly, great for brands looking to appear approachable.
- Spanish (Latin America): Used for content tailored to the Latin American market.
In some cases, it’s also important to adapt the accent to reflect local dialects or variations within a country, ensuring that the voice feels authentic and is well-understood by the audience.
Note: Customization options for both tone and accent can typically be fine-tuned through advanced machine learning models, which allow the developer to balance between a natural-sounding voice and the desired characteristics for each use case.
Summary Table
Use Case | Preferred Tone | Common Accents |
---|---|---|
Customer Service | Empathetic, Professional | Neutral American, British |
Entertainment | Casual, Excited | Neutral American, Australian |
Instructional Content | Professional, Authoritative | Neutral American, British |
Understanding the Limitations and Ethical Considerations of Deep Fake Voices
Deep fake voice technology, while revolutionary in many ways, is not without its limitations. The accuracy and quality of synthesized voices heavily depend on the quantity and quality of the source material. Inconsistent audio input or insufficient data may result in robotic or unnatural speech patterns, undermining the believability of the deep fake voice. Moreover, the complexity of human emotions, tone, and subtle nuances can be challenging for current algorithms to replicate convincingly.
Beyond technical constraints, there are critical ethical concerns that arise with the use of deep fake voices. Misuse of the technology for malicious purposes, such as impersonating individuals for fraud or creating misleading media, is a growing risk. These ethical issues demand careful consideration and regulation to prevent harm and exploitation. The broader societal impact of deep fake voices includes the potential erosion of trust in audio-visual content, as people become increasingly skeptical about the authenticity of what they hear.
Limitations of Deep Fake Voice Technology
- Data Dependency: Deep fake voices require large, high-quality datasets to produce convincing outputs. Insufficient data or low-quality recordings can lead to errors in voice synthesis.
- Emotional Complexity: Capturing nuanced emotions and intonations in speech remains a challenge. Machines still struggle with replicating the full spectrum of human vocal inflections.
- Contextual Relevance: Deep fake voices may struggle to maintain consistency in complex conversational contexts, often failing to adapt appropriately to changing topics or emotional shifts.
Ethical Risks and Concerns
Ethical use of deep fake voice technology requires responsibility and transparency to ensure it does not harm individuals, spread misinformation, or erode trust in media.
- Impersonation and Fraud: Criminals can exploit deep fake voices to impersonate individuals, leading to identity theft, financial fraud, and other malicious acts.
- Spread of Misinformation: Fabricated audio can be used to mislead the public, manipulate opinions, or deceive audiences by creating fake statements attributed to public figures.
- Loss of Trust: The proliferation of convincing deep fake voices could lead to a broader skepticism about the authenticity of audio recordings, damaging trust in legitimate sources.
Key Considerations for Ethical Use
Consideration | Description |
---|---|
Transparency | Clear labeling of deep fake content is essential to prevent deception and ensure accountability for its creation. |
Consent | Obtaining explicit consent from individuals whose voices are being used for deep fake synthesis is a necessary ethical safeguard. |
Regulation | Governments and organizations must implement regulations to restrict the misuse of this technology while encouraging its positive applications. |
How to Integrate AI-Generated Voices into Your Marketing Campaigns
With the rise of deepfake voice technology, businesses now have the opportunity to enhance their marketing strategies through highly realistic voice synthesis. This approach enables companies to create engaging, personalized experiences without the need for traditional voice actors or expensive recording sessions. By leveraging AI-generated voices, brands can deliver dynamic audio content that speaks directly to their audience’s preferences, all while maintaining a consistent and professional tone across multiple channels.
When done correctly, deepfake voice technology can add a new layer of immersion and emotional connection with your audience. Here’s how you can incorporate this cutting-edge tool into your marketing campaigns:
1. Personalize Customer Interactions
AI-generated voices allow you to create highly personalized interactions with your customers. For example, using a deepfake voice, you can deliver tailored messages during customer service calls or automated responses. This can make the interaction feel more human and engaging, leading to better customer satisfaction.
- Use AI-generated voices for personalized phone calls.
- Implement it in interactive voice response (IVR) systems for dynamic customer support.
- Create unique ad campaigns with voices tailored to different demographic segments.
2. Enhance Brand Storytelling
AI voices can help bring your brand’s story to life in an engaging and memorable way. Instead of relying on the same static voiceover for all your videos or ads, deepfake technology allows you to experiment with various voices that reflect different moods and personalities, giving your content a more immersive quality.
- Develop a voice persona for your brand and apply it across different media formats.
- Use voice AI to narrate stories, podcasts, or video campaigns that align with your brand’s values.
- Test multiple voices for A/B testing in ads to see which one resonates best with your audience.
3. Scale Your Audio Content Production
Creating audio content at scale can be time-consuming and expensive. With deepfake voice technology, you can produce high-quality voiceovers quickly and affordably. This is particularly useful for companies that need to create multiple versions of content for different regions, languages, or market segments.
Benefit | Impact |
---|---|
Speed | Generate large amounts of content in a short time. |
Cost Efficiency | Avoid high costs associated with hiring voice actors for every project. |
Localization | Produce content in multiple languages or accents effortlessly. |
“Deepfake voice technology enables businesses to scale their audio content production, making it easier to experiment with different messaging styles and audience targeting without the logistical challenges of traditional voiceovers.”
Real-World Applications of Voice Synthesis in Customer Support
In recent years, artificial intelligence has revolutionized customer service by providing innovative solutions for improving user experience and operational efficiency. One such breakthrough is the integration of voice synthesis technology, commonly known as deep fake voice creation. This tool allows businesses to replicate human-like voices, providing a new dimension in customer support. By mimicking a range of vocal tones and inflections, these synthetic voices are making customer interactions more natural and personalized.
Deep fake voice technology has a variety of uses in customer service, from handling routine inquiries to offering a more personalized touch in client interactions. By utilizing synthesized voices, businesses can cut down on the need for live agents, reduce wait times, and improve overall customer satisfaction. Moreover, the potential for multilingual support opens up new opportunities for global service delivery without the logistical challenges of hiring multilingual staff.
Key Benefits of Voice Synthesis in Customer Support
- Cost-Effectiveness: Reduces the need for large customer service teams, especially for handling repetitive queries.
- 24/7 Availability: Allows businesses to provide round-the-clock support without the constraints of human scheduling.
- Enhanced User Experience: Mimics natural speech patterns, creating more engaging and human-like interactions.
- Multilingual Capabilities: Offers seamless interaction in different languages, improving accessibility for a global customer base.
Applications in Real-World Scenarios
- Automated Customer Service Calls: Voice synthesis can handle basic customer queries such as account balances or order status without human intervention.
- Personalized Greetings and Follow-Ups: Businesses can use deep fake voices to send personalized messages or reminders, making customers feel more valued.
- Training and Simulation: Virtual agents can simulate conversations for training purposes, helping new employees familiarize themselves with customer interactions.
"By integrating synthetic voices, businesses can deliver consistent and scalable customer service experiences that retain a high level of personalization."
Comparing Deep Fake Voice Technology vs. Traditional Customer Service
Aspect | Traditional Service | Synthetic Voice Service |
---|---|---|
Response Time | Varies, typically slow during peak hours | Instant, 24/7 availability |
Cost | Requires hiring and training agents | Lower operational costs, no need for staff on call |
Personalization | Limited by agent capacity and training | Highly customizable based on customer profile |